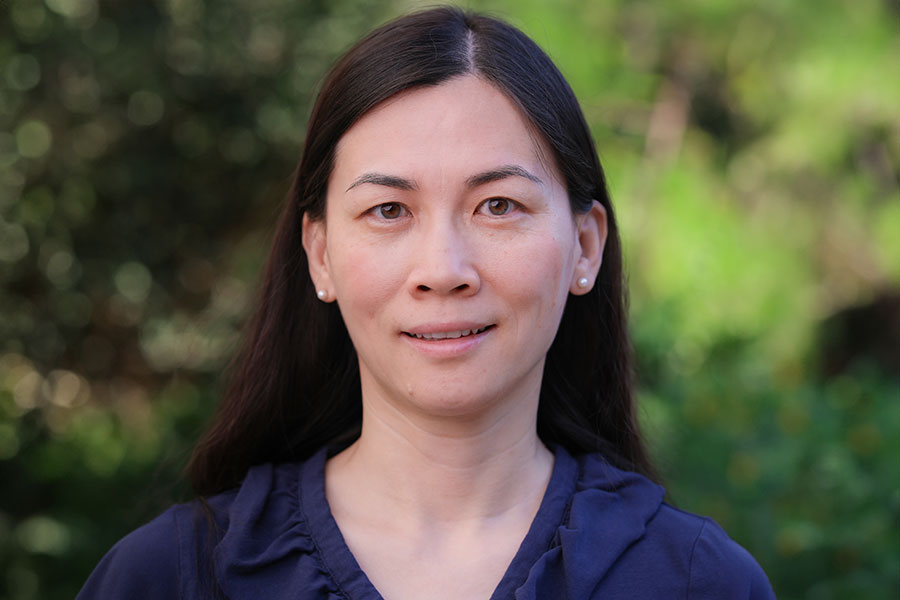
A Florida State University researcher has been elected as a fellow of the American Statistical Association for her outstanding contributions to the field of statistics.
Professor of Statistics Hongyuan Cao was recognized by ASA for her research in high-dimensional statistics, longitudinal data analysis and survival analysis, with a strong emphasis on applications in social science, biological science and medical science.
“I am excited and feel very honored,” Cao said. “By validating my past work, the fellowship enhances the visibility and credibility of my ongoing and future research.”
Cao is among 47 ASA fellows selected worldwide this year in recognition of their professional contributions, leadership and commitment to the field of statistics. Founded in 1839, ASA is the world’s largest community of statisticians and second-oldest continuously operating professional association in the U.S.
“The fellowship is a prestigious recognition of Cao’s excellence in research, teaching, and service to the statistical community,” said Eric Chicken, professor and chair of the Department of Statistics. “It also acknowledges the high-quality work conducted within the department and highlights FSU’s role as a leading institution for statistical research and academic excellence.”
One of Cao’s research areas is on causal inference. She redefines heritability, a concept central to social, biological and medical sciences, from the causal inference perspective.
Cao investigates the missing heritability puzzle, where there is a substantial gap between heritability estimated from twin studies and population-based cohort studies. While genetics are normally set by the time a person is born, lifetime interactions with different drugs and chemicals and individuals’ lifestyles are among the external factors that can turn certain genes “on” or “off.” Better understanding the interplay between a person’s genetics and their environment has far-reaching implications for the personalization and effectiveness of health care.
“If one group or demographic is more likely to inherit a certain trait, that information can be incorporated into clinical trials to develop more effective screening and disease management strategies,” Cao said. “It can also improve the precision with which specific populations are identified as having higher genetic risk for certain diseases.”
Cao also uses statistical analysis to evaluate the replicability of past studies to improve the structure of future studies. A study is considered replicable when the same conclusions can be drawn at different times, locations and populations. The scientific method hinges upon replicability, as it validates research results.
“Replicability is the cornerstone of modern scientific research, and it has recently attracted considerable attention, scrutiny, and debate in both the popular press and scientific community,” Cao said. “Having replicable findings provides stronger evidence, avoids wasted resources, and improves the efficiency of drug development. This helps the translation of benchmark discoveries into bedside therapies, improving human health.”
Of the several categories that may cause non-replicability, Cao focuses on data analysis and reporting. In particular, she studies conceptual replicability, where consistent results are obtained using different processes and populations that target the same scientific question.
“Cao’s work is characterized by the development of innovative statistical methodologies and their practical applications to solve complex, real-world problems,” Chicken said. “Her diverse teaching experience underscores her commitment to advancing the field and supporting the next generation of statisticians.”
Cao also dedicates time to longitudinal data analysis and survival analysis, for which she investigates a particular dataset of COVID-19 patients. She examines data that is repeatedly recorded over a period of time, including vital signs during a hospital stay. Survival analysis is a statistical method used to analyze the time it takes for a particular event to occur. In Cao’s research, this event of interest is each patient being discharged. A better prediction of hospital stay allows for more effective resource allocation and disease management.
“Statisticians and data scientists often play an invisible role in research, but it is an invaluable one,” Cao said. “The ASA fellowship provides a platform for greater collaborations both within and outside of the statistical community, which leads to more significant advancement and innovation in all fields.”
For more information about statistics and data science research at FSU, visit stat.fsu.edu.